The Model Context Protocol (MCP) is one of the latest advancements in the field of Agentic AI. Originally developed by Anthropic and subsequently open-sourced, MCP establishes a standardized framework that enhances AI assistants' capabilities by enabling them to interact with external data sources and tools. This protocol essentially allows AI systems to extend beyond their built-in knowledge and connect with the broader digital ecosystem.
Despite being less than six months old, MCP has gained remarkable traction within the AI community. The rapid adoption rate has accelerated ecosystem development, creating both opportunities and security challenges that organizations must understand before implementation.
A General View of MCP's Architecture
The MCP protocol defines 5 entities that it connects:
- MCP Hosts: Applications that use MCP to access data, like Claude Desktop or other AI tools
- MCP Clients: Components that connect directly to servers using the protocol. For most people, the Host and Client are a single black box component.
- MCP Servers: Simple programs that make tools available to hosts through MCP
- Local Data Sources: Data or services on the host's computer that MCP servers can access
- Remote Services: External systems, typically web APIs, that MCP servers can connect to
There is, in general, an additional component with the LLMs themselves that are remotely accessed via an AI provider API.
The general behavior of a system relying on this architecture is as follows:
- The user prompts the host for a task.
- The host and its bound LLM will decide if it needs additional capabilities to perform the task.
- If a tool is needed, the host will send a request to the appropriate server, for example, to access the content of a file on the filesystem.
- The server runs the tool it provides (running a system command or an API call) based on inputs from the LLM thinking process and sends the result of the execution back to the host.
- With the information back from the tool, the host (and LLM) can finish its thinking process and provide an answer to the user's prompt.
For example, when a financial analyst asks an AI assistant to "analyze Q1 earnings for tech companies compared to expectations," the host might request financial data from a remote MCP server connecting to a market database, then utilize a local MCP server to generate visualization charts, all while the LLM orchestrates these components to deliver a comprehensive analysis that would be impossible with the base model alone.
This process is, in essence, similar to what has existed with microservices for years, and the concept of standalone, purpose-built, reusable servers similar to Docker images. The difference here is that an AI is in charge of the components' orchestration.
An Introduction to MCP's Threat Model
The main new components brought by the MCP protocol are the MCP servers. There are two kinds of servers:
- Local servers: Which provide access to local data and services like local file read, or command line execution
- Remote servers: Which give access to remote resources: data in a database, remote API, etc.
For example, a local MCP server might provide access to a user's file system, allowing an AI assistant to read requested documents or save generated content. Meanwhile, a remote MCP server might connect to a company's customer database, enabling the AI to retrieve specific customer information when needed for analysis.
While local servers are naturally meant to be hosted on the same machine as the MCP host, remote servers can be deployed locally or on a distant host. This distinction fundamentally changes the threat model for the MCP architecture.
Local MCPs
Local MCP servers provide access to sensitive resources such as the file system or command shell. The primary risk stems from exposing these resources to the MCP client. When LLMs gain access to local resources, they become potential vectors for system compromise if they receive malicious instructions.
These harmful instructions might originate from various sources: prompt injections on publicly accessible MCP hosts, model hallucinations, poisoned training data, or even from the LLM provider itself if they have malicious intent.
Additionally, if MCP client credentials are compromised, the presence of local MCP servers increases the potential impact of such a breach, as attackers could leverage these credentials to access sensitive local resources through the authorized MCP connection.
Local MCP servers can also introduce privilege escalation vulnerabilities if they fail to properly authenticate and authorize their users. For instance, a local MCP server running with elevated system privileges but implementing weak authentication could allow a standard user to execute commands with higher privileges than they normally possess. This creates a dangerous security gap where users could potentially access or modify sensitive system files, install malware, or perform other unauthorized actions with escalated permissions.Remote MCPs
Remote MCP servers connect to external services and require authentication credentials to function. Rather than embedding these secrets directly in the server (a poor security practice), they must be provided through configuration.
This creates multiple security concerns. First, the configuration containing sensitive credentials must be transmitted over the network, increasing exposure through potential network interception, logging by infrastructure components, and sharing with both the server owner and hosting provider (which may be different entities).
Moreover, the use of remote MCP servers expands the attack surface for credential exposure and potentially increases the proliferation of sensitive information across systems.
The Secrets of an MCP Architecture
The multiplication of small independent components in an MCP architecture is an important cause of Non-Human Identities' proliferation. There are at least two kinds of secrets that are necessary for any MCP-based application to function:
- LLM API credentials: They allow the MCP host to communicate with its AI provider’s API
- Remote services credentials: Remote MCP servers usually require credentials to access a remote API or authenticate the client against a remote data source.
While there will usually be only one identity to authenticate the host to the AI provider API, there is at least one for every remote server deployed in the architecture. This can already represent a vast number of secrets, but they will generally be joined by other, non-strictly necessary ones:
- MCP server authentication credentials: In some cases, MCP servers need to be restricted to authorized users only.
- OAuth credentials: The MCP specification defines an authorization model based on OAuth. This model requires additional OAuth secrets.
- MCP server certificates: Remote servers are usually accessed over the network. Doing so securely, using a TLS-based security layer, requires server certificates and keys.
Each of these secrets is at risk of being leaked, with various consequences depending on its type. Indeed, while leaking LLM API and Remote services credentials will generally lead to common consequences (increased services costs, sensitive data access, etc), a compromised MCP server certificate’s private key can prove even more dangerous. Given the connection between all the components of the MCP architecture, attackers who can impersonate an MCP server would be able to target the deployment’s local components: the host and the local MCP servers. This would open the door to prompt injection in critical local servers with potential local host compromise.
Analyzing MCP Servers’ Secrets
MCP servers are, by nature, meant to be shared across the MCP user community. For this reason, MCP server registries are already starting to be created. They are meant to provide a similar experience to DockerHub for Docker images and, thus, host a wide variety of community-submitted MCP servers.
Smithery.ai is one of those registries. It currently provides access to more than 5,000 MCP servers, which are backed by GitHub code repositories. The GitGuardian security research team analyzed those to provide insight into the state of secrets sprawl in the MCP servers ecosystem.
From the 5,000 available servers, 3829 public GitHub repositories were cloned. Other servers are either not public yet or rely on a private repository. The scan of those repositories revealed that 202 of them leaked at least one secret. That’s 5.2% of all the repositories, a number that is slightly higher than the 4.6% occurrence rate observed on all public repositories. While this number is not significantly higher than expected, it still illustrates how MCP servers are a new source of secret leaks.
Interestingly, the research found that the secret incidence rate on repositories does not change depending on the type of server, remote or local, with both rates set around 5.2%. In fact, the leaked secrets type does not differ significantly between the types of servers.
Regarding secret types, setting aside the generic secrets about which not much can be said, the analysis revealed a high number of Bearer tokens and X-Api-Key, which are common for API authentication. This is not surprising considering the role of MCP servers and tends to confirm that those represent a critical new surface of secret exposure.
Beware of the MCP Security Environment
MCP is definitely an exciting new protocol that further enhances the capabilities of modern AI assistants. However, it comes with added security risks that should be taken into account before organizations move forward with this technology. Whether developing MCP servers or simply using them, there are important security considerations to address.
For servers’ developers, one of the main points is to properly manage remote services authentication. If, as with any piece of software, the secrets to the remote services should not be embedded in the server’s source code, in this case, the server should not be the owner of the secret at all. Instead, the clients should provide the appropriate secrets when querying the server. This means that server developers are also responsible for the proper handling of those secrets:
- The servers should only be available through a secure communication channel (such as TLS).
- The server should not store the clients' secrets.
- When logging information, the server should make sure no secrets are exposed.
- More generally, the servers should be developed with security in mind, as a compromise of one server could lead to the exposure of all clients’ secrets. Proper user input validation is a must in that context.
For the MCP protocol users, the main point is to understand the added risks and take action accordingly. The main point to consider in an MCP risk assessment is that this technology opens communication channels from remote servers (sometimes on the internet) to local machines with direct access to sensitive capabilities. This is a risk that organizations can either accept or mitigate. If accepted, proper hardening measures should be implemented:
- Enforce manual approval before any sensitive action by the MCP host.
- Restrict the commands LLMs can run at the MCP level.
- Consider containerizing sensitive local servers when that is possible.
And, of course, organizations should protect their client secrets and implement proper secret detection measures to identify potential exposures before they can be exploited. It is worth noting that MCP servers and architectures mostly rely on code hosted on traditional code hosting platforms. Detecting secrets in those environments is a classic issue that is already tackled by GitGuardian’s secret detection solutions.
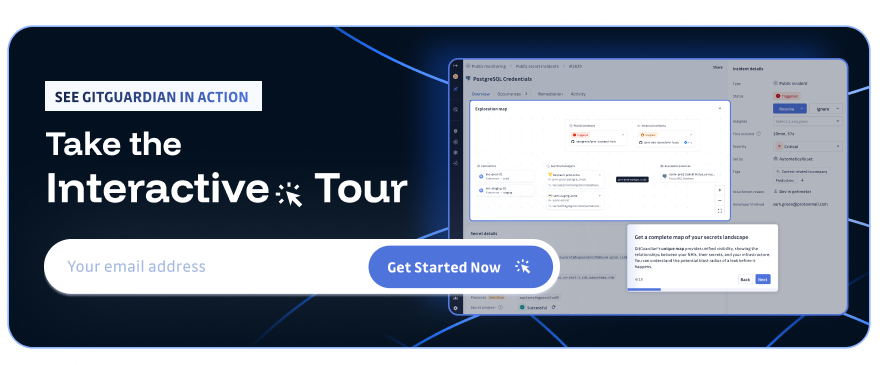